Research
Our Research Areas
Our current direction is towards researching and developing AI-integrated solutions for vibration control, structural health monitoring, and modal testing that are practical for industrial applications. Some of our main research areas are modal analysis, structural damage diagnosis, fault diagnosis, force identification (inverse problem), and energy harvesting.
Modal Analysis
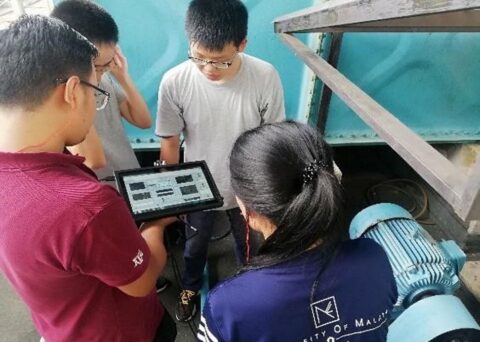
Our team has developed and alternative modal analysis techniques, i.e., Impact-Synchronous Modal Analysis (ISMA) that can be performed on operating structures during measurement. Through impact-synchronous time averaging (ISTA), the harmonics and noise in the signals can be removed, thus producing clean modal data. Recent developments include combining human behaviour recognition to improve the efficiency and accuracy of the ISMA method.
Force Identification (Inverse Problem)
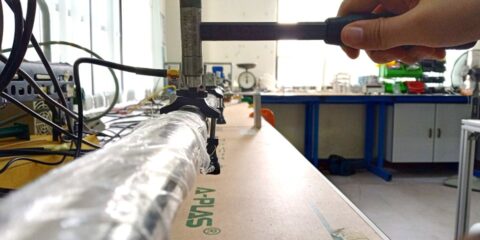
Impact force identification is the process of estimating the force that is applied to a structure or object as a result of an impact event. This is typically done by analyzing the response of the structure or object to the impact, such as the acceleration or displacement of the object, and using this information to infer the force that was applied. Impact force identification is commonly used in a variety of fields, including structural engineering, automotive safety, and sports equipment design, to better understand the effects of impacts and to design structures and objects that can withstand or mitigate the forces generated by impacts.
Structural Damage Diagnosis
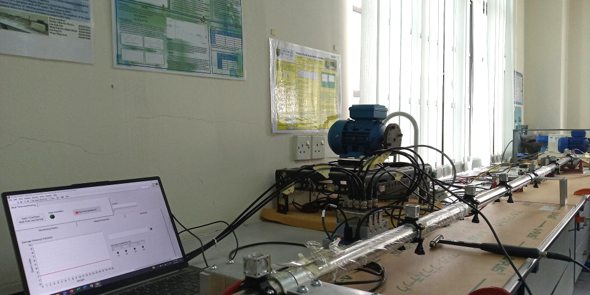
Damage diagnosis is an important step in structural health monitoring. Machine learning-based damage diagnosis methods are extensively research in the recent decade. Our team has investigated both unsupervised and supervised machine learning methods for structural damage diagnosis. Recent research includes investigating an interesting strategy by integrating modal-based and machine learning methods to solve the cold-start issue faced by machine learning methods. Developing a hybrid digital twin is an ongoing research that aims to extend the current method towards damage prognosis.
Fault Diagnosis
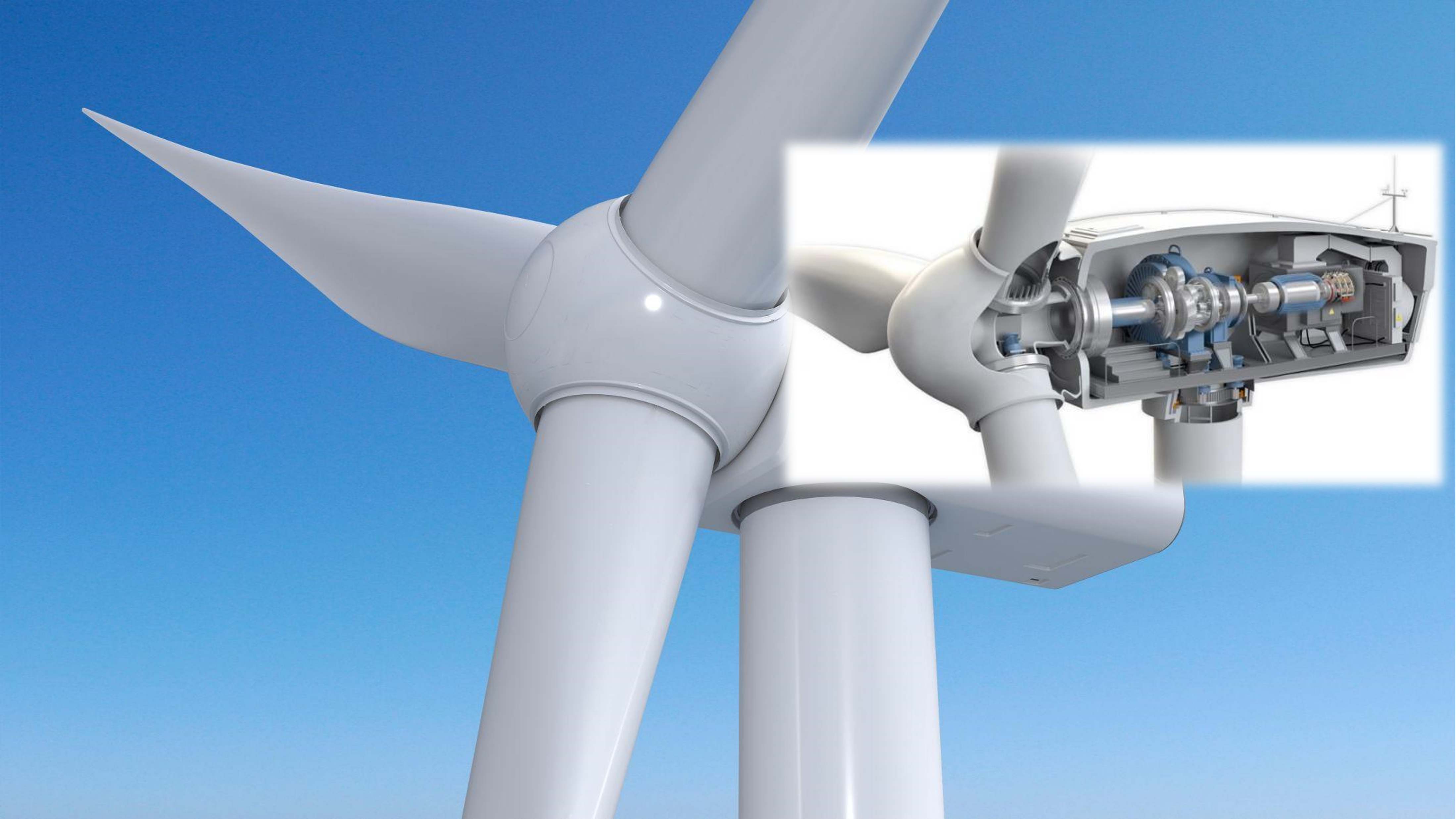
Fault diagnosis primarily deals with identifying and characterizing deviations or abnormalities in the operational performance of a structure or system. Our team is currently investigating deep learning methods and entropy measures for fault diagnosis of wind turbine systems. Deep learning methods utilize artificial neural networks with multiple layers to learn complex patterns and relationships from large datasets, enabling automated fault detection and diagnosis in wind turbines. Entropy measures provide a quantitative assessment of the randomness or disorder within a signal, and by applying improved entropy measures, it becomes possible to analyze the gearbox’s vibration signals and identify potential faults.
Energy Harvesting
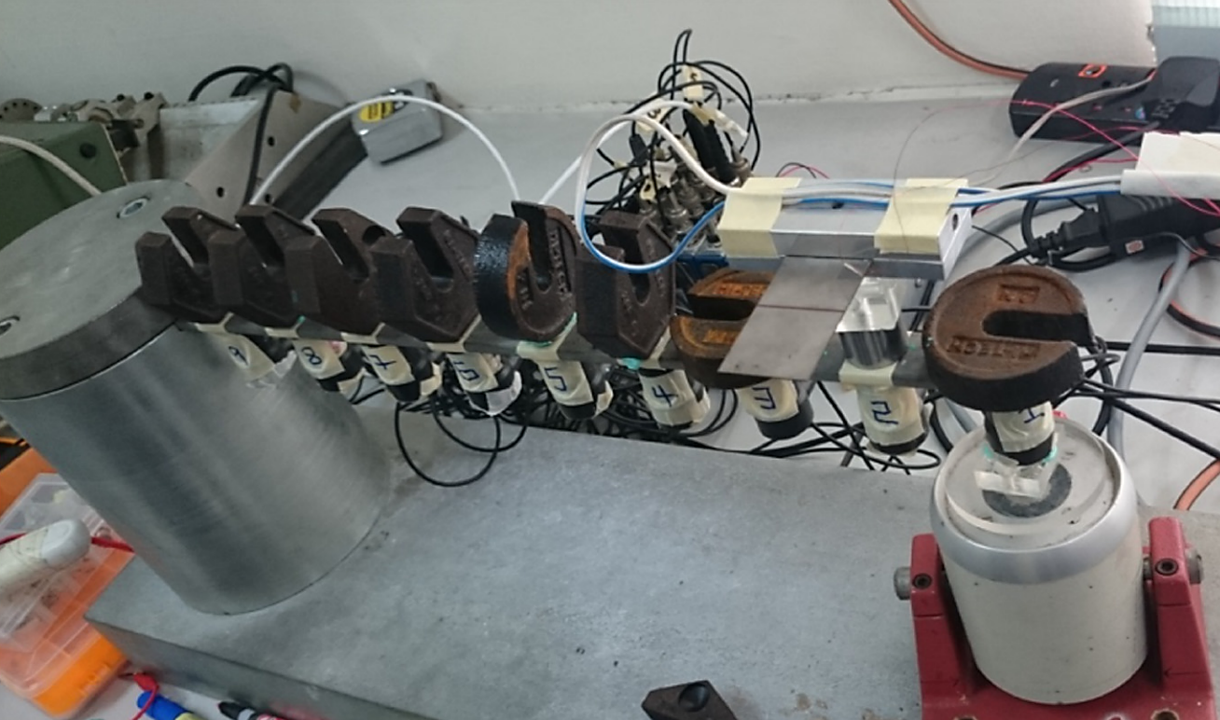
An energy harvester is a device that captures energy from the surrounding environment and converts it into electrical energy that can be used to power electronic devices. Energy harvesters are also known as energy scavengers or power harvesters. They can be used in a variety of applications, including wireless sensor networks, Internet of Things (IoT) devices, wearable electronics, and medical implants.