Resesarch
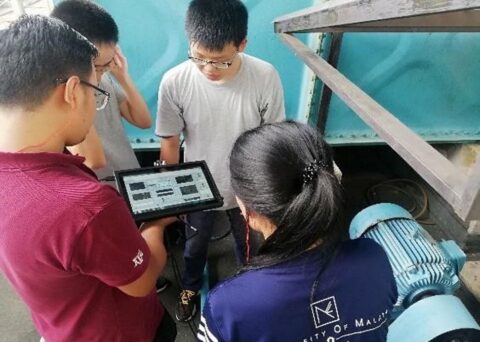
Impact-Synchronous Modal Analysis
Impact-Synchronous Modal Analysis is an alternative modal analysis technique that can be performed on operating structures. Through impact-synchronous time averaging (ISTA), the harmonics and noise in the signals can be removed, thus producing clean modal data.
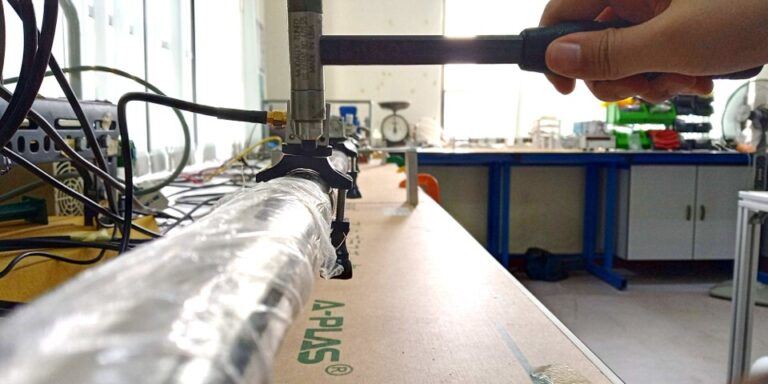
Force Identification
Impact force identification is the process of estimating the force that is applied to a structure or object as a result of an impact event. This is typically done by analyzing the response of the structure or object to the impact, such as the acceleration or displacement of the object, and using this information to infer the force that was applied. Impact force identification is commonly used in a variety of fields, including structural engineering, automotive safety, and sports equipment design, to better understand the effects of impacts and to design structures and objects that can withstand or mitigate the forces generated by impacts.
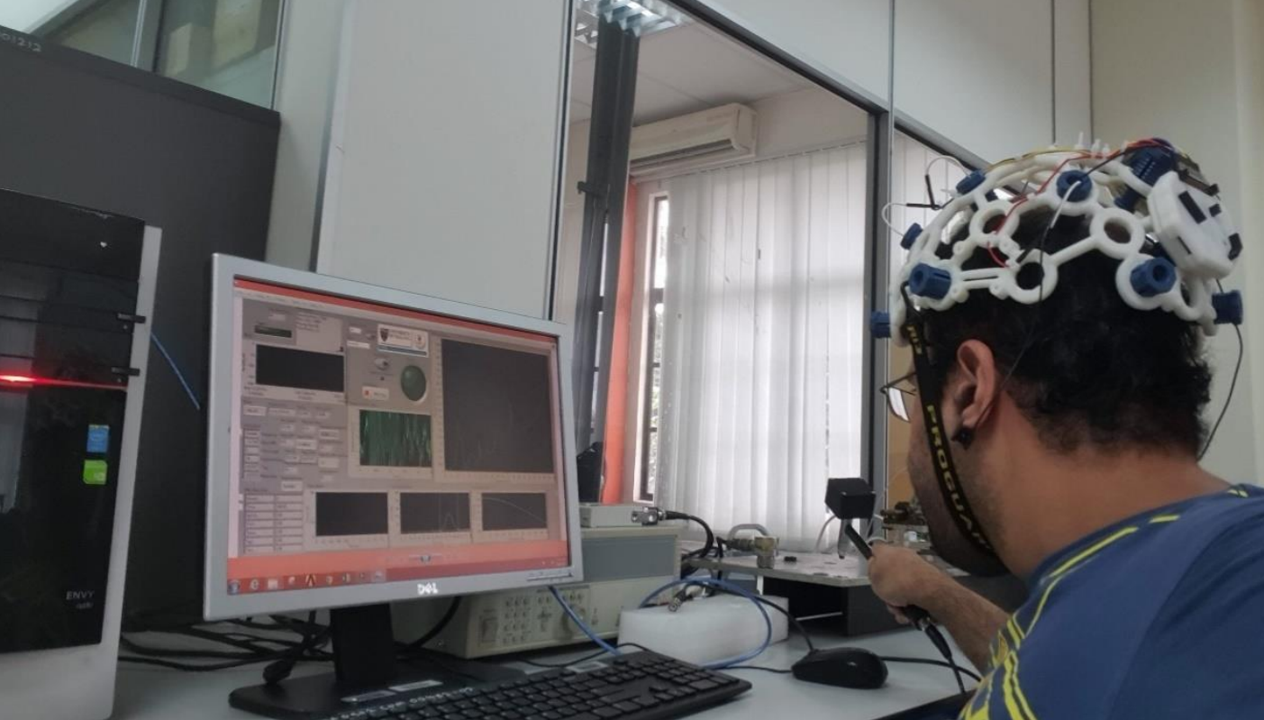
Semi-Automated Impact Device for ISMA
As the practicality and effectiveness of ISMA is dependent on the impact control, this research investigates the relationship between human brain waves and the reaction time for impact to develop a semi-automated impact device. Machine learning techniques and a low cost, portable and wireless Electroencephalogram (EEG) device are integrated to build the semi-automated device, or also known as EEG-ISMA.
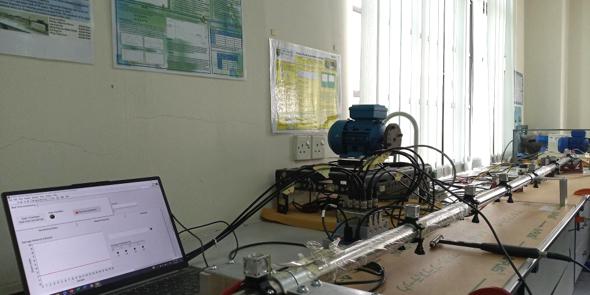
Hybrid Modal-Machine Learning Approach for Structural Damage Diagnosis
Machine learning-based damage diagnosis method are extensively research in recent decades. This research investigates an interesting strategy by integrating modal-based and machine learning methods to solve the cold-start issue, i.e., limited training samples for machine learning methods.
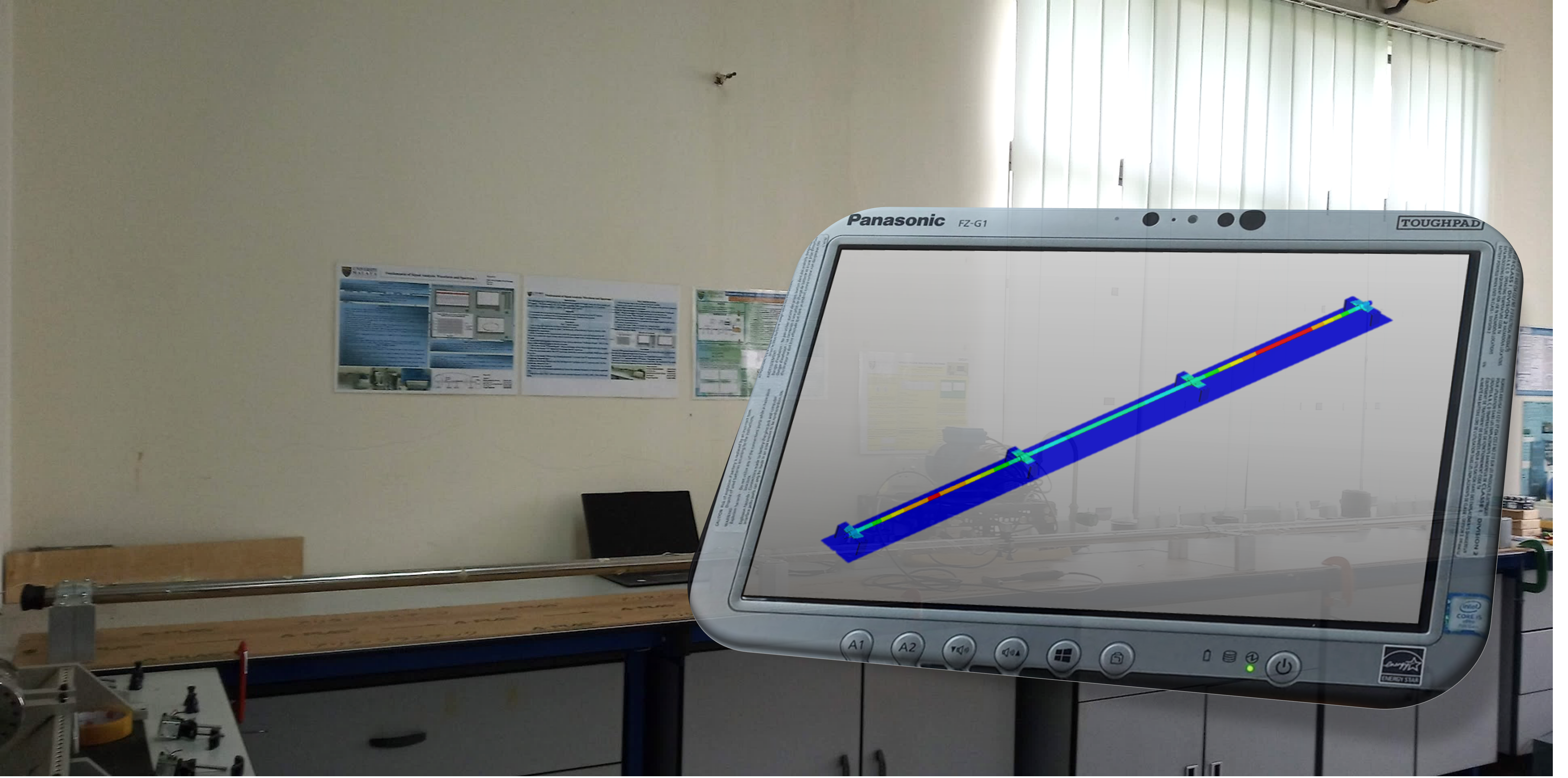
Hybrid Digital Twin for Predictive Maintanence
In a hybrid digital twin for predictive maintenance, a digital twin is created for a physical asset, such as a machine or piece of equipment. This digital twin is connected to the physical asset through sensors and other data sources, allowing it to gather real-time data on the asset's condition and performance. This data is then analyzed using predictive maintenance techniques to identify patterns and anomalies that could indicate potential issues or failures.
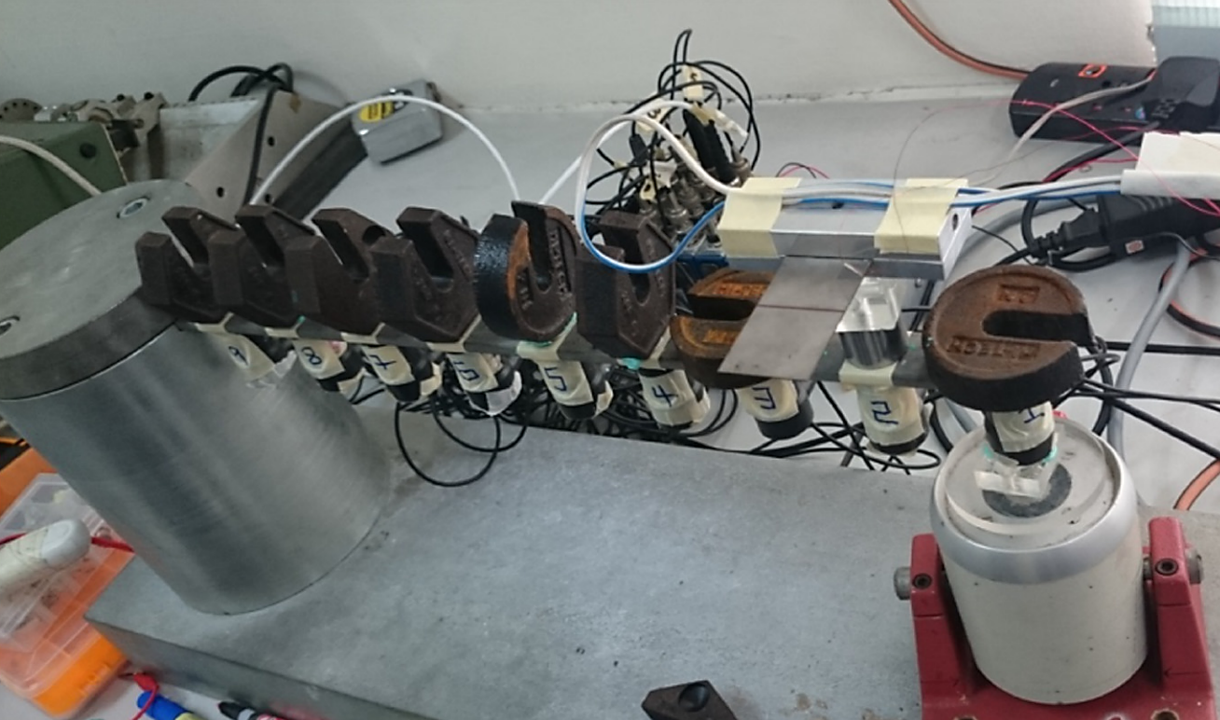
Energy Harvester
An energy harvester is a device that captures energy from the surrounding environment and converts it into electrical energy that can be used to power electronic devices. Energy harvesters are also known as energy scavengers or power harvesters. They can be used in a variety of applications, including wireless sensor networks, Internet of Things (IoT) devices, wearable electronics, and medical implants.
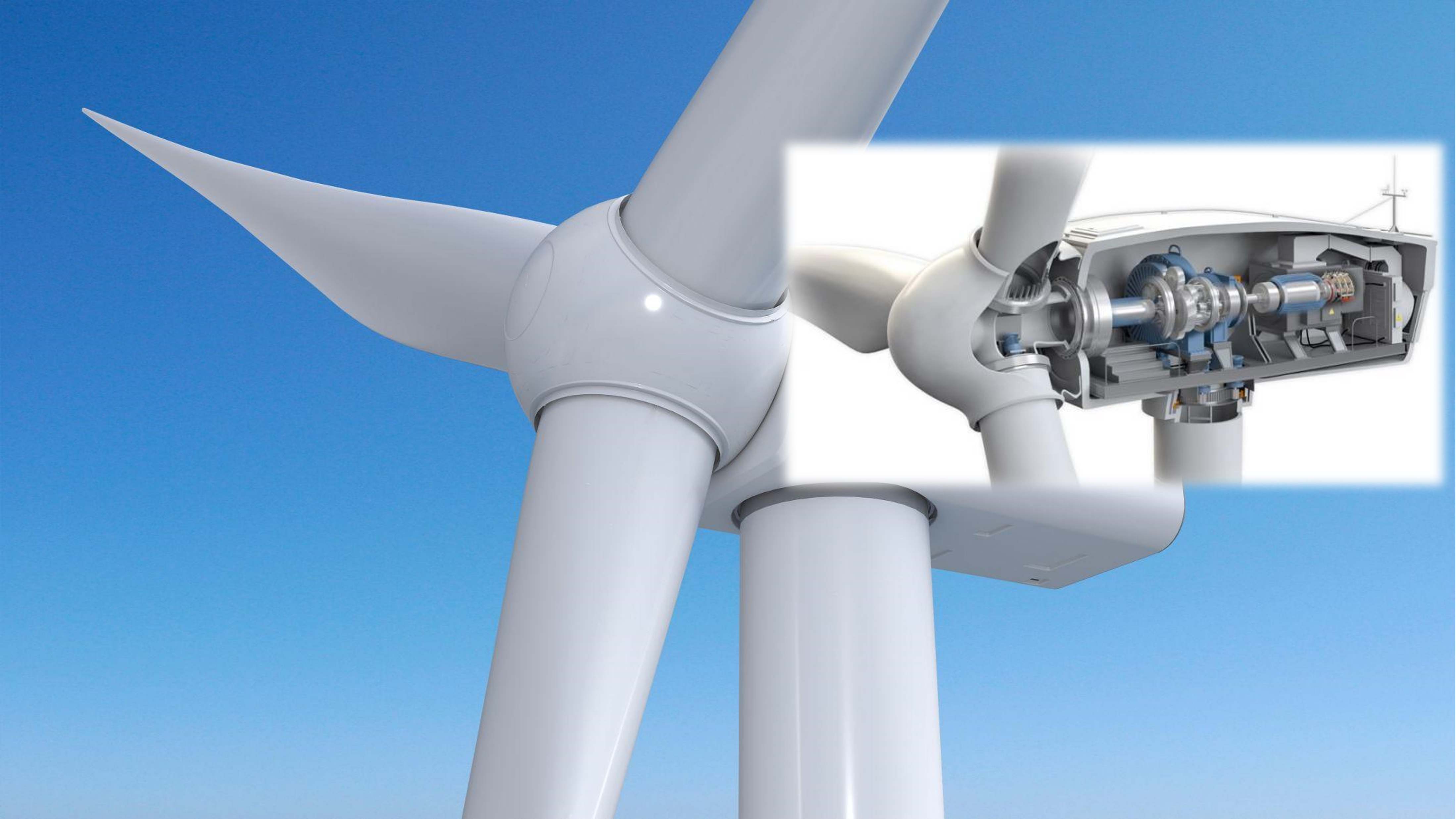
Fault Diagnosis of Wind Turbine Gearbox using Improved Entropy Measures
Fault diagnosis of wind turbine gearboxes using improved entropy measures refers to a technique that utilizes entropy-based measures to detect and diagnose faults or abnormalities in the gearbox of a wind turbine. Entropy measures provide a quantitative assessment of the randomness or disorder within a signal, and by applying improved entropy measures, it becomes possible to analyze the gearbox's vibration signals and identify potential faults.
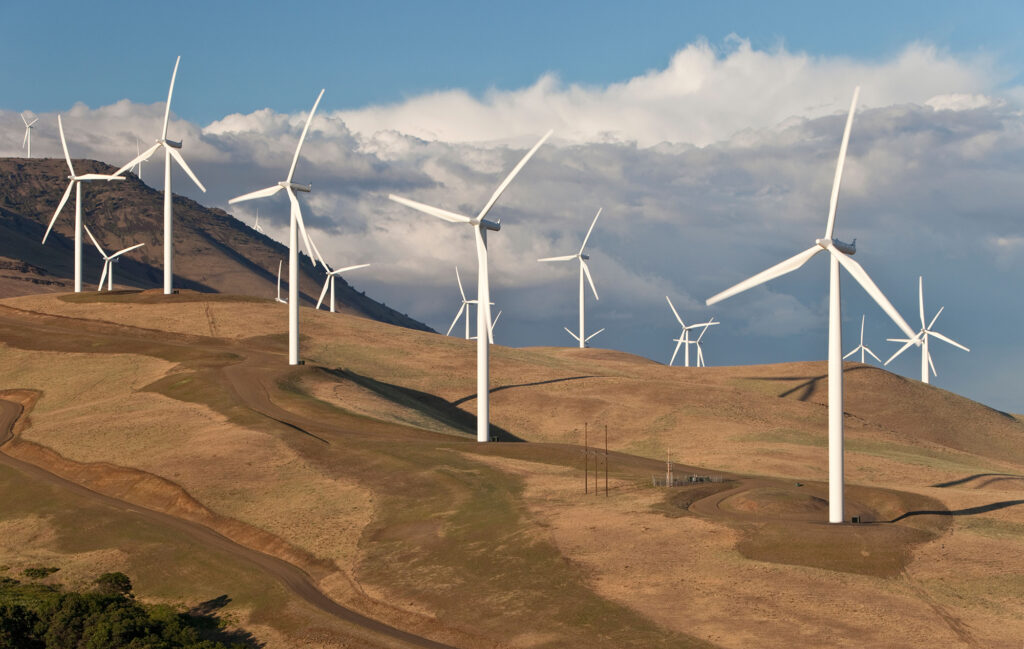
Fault Diagnosis for Wind Turbine Systems using Deep Learning Methods
Fault diagnosis for wind turbine systems using deep learning methods involves the application of advanced machine learning techniques, specifically deep learning algorithms, to detect and diagnose faults in wind turbine systems. Deep learning methods utilize artificial neural networks with multiple layers to learn complex patterns and relationships from large datasets, enabling automated fault detection and diagnosis in wind turbines.